Mastering Labeling Training Data for Enhanced AI Accuracy
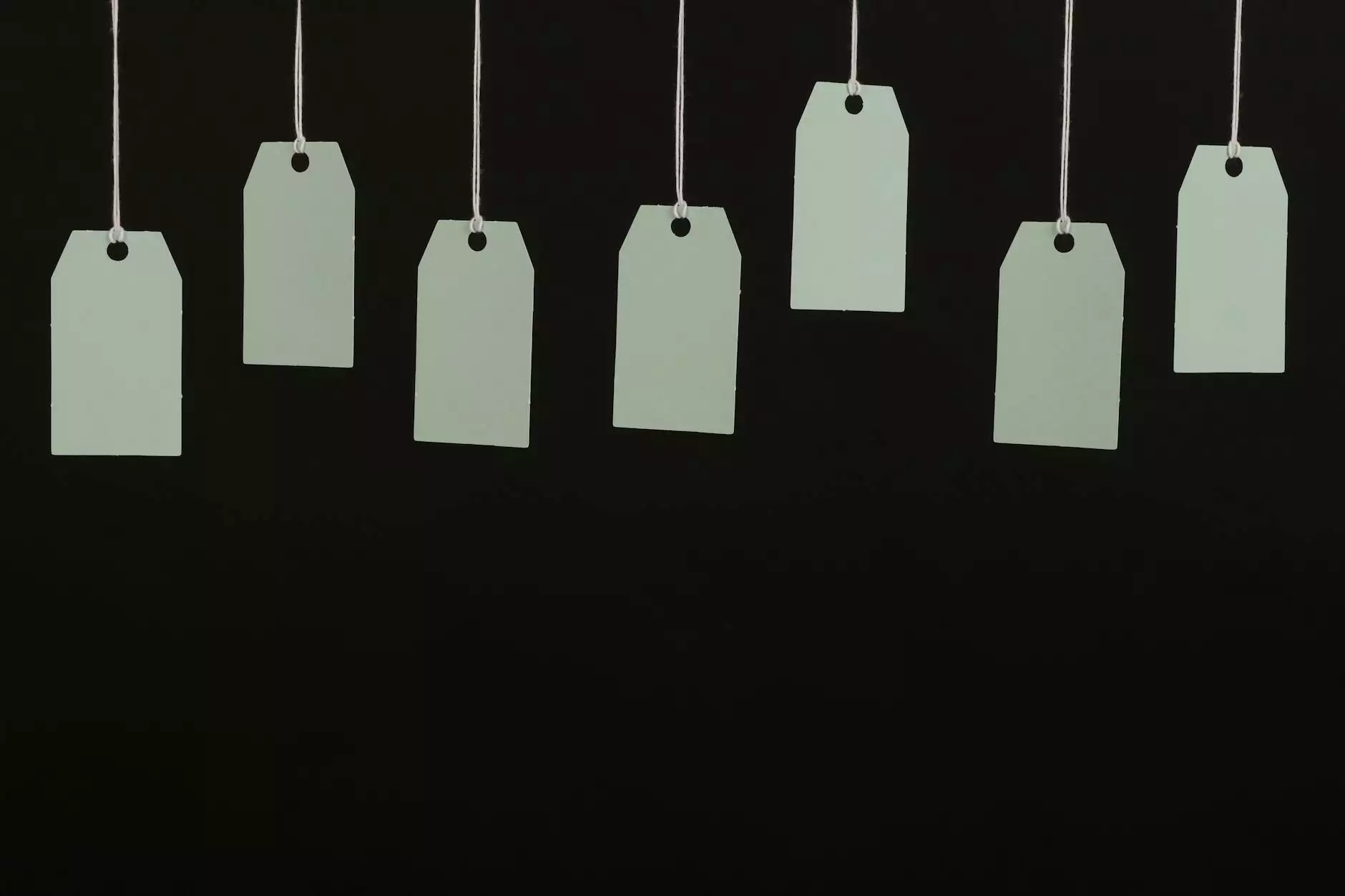
In the rapidly evolving world of artificial intelligence (AI), one of the critical components that drive the performance of AI models is labeling training data. The process is essential for teaching machines how to interpret data accurately, making it a cornerstone of effective machine learning and deep learning solutions. In this article, we will delve into the intricacies of labeling training data, its significance, and how using comprehensive data annotation tools and platforms can dramatically enhance AI performance.
Understanding Labeling Training Data
Labeling training data involves the systematic process of tagging and categorizing data with labels that define what the data represents. This process is fundamental for supervised learning, where the model learns from the labeled data. Without accurate and high-quality labels, AI models can produce unreliable or biased results.
The Importance of Data Labeling
There are several reasons why labeling training data is pivotal in machine learning:
- Model Performance: Well-labeled data directly influences the accuracy and reliability of AI models.
- Bias Reduction: Proper labeling reduces the likelihood of bias by ensuring diverse and accurate representation within the training data.
- Training Efficiency: High-quality labels shorten the time required for the model to learn, as they provide clear guidance during the training process.
- Improved Predictions: Accurately labeled data allows AI systems to make more precise predictions, leading to better outcomes in applications.
Types of Labeling in Training Data
Labeling training data can take several forms, depending on the nature of the data and the intended application. Below are some common types of labeling:
1. Image Annotation
Involves tagging images with relevant information. Common tasks include:
- Bounding Boxes: Enclosing specific objects within a larger image.
- Segmentation: Classifying each pixel in an image to create more detailed understandings of the scene.
- Landmark Identification: Marking key points on objects in the images.
2. Text Annotation
This involves labeling text data, which can include:
- Named Entity Recognition (NER): Identifying and classifying entities in text into predefined categories.
- Sentiment Analysis: Assessing the sentiment expressed in textual data.
- Text Classification: Categorizing text based on its content.
3. Audio and Speech Annotation
Sound data can also be labeled for various applications:
- Speech Transcription: Converting spoken language into written text.
- Emotion Recognition: Labeling audio clips based on the emotions expressed.
- Sound Event Detection: Identifying specific sounds within audio recordings.
The Process of Labeling Training Data
To ensure effective labeling training data, it is crucial to have a well-defined process. This typically involves the following steps:
1. Data Collection
Gathering raw data from various sources is the first step. The data must be representative of the problem domain to ensure comprehensive training for the AI model.
2. Data Cleaning
Raw data often contains noise and irrelevant information; thus, cleaning the data is necessary to improve labeling accuracy.
3. Annotation Guidelines Development
Creating clear guidelines helps ensure that the labeling process is consistent and that multiple annotators can produce uniform results.
4. Manual and Automated Labeling
Data can be labeled manually by teams of annotators or through automated systems using algorithms. Each approach has its pros and cons, depending on the complexity and volume of data.
5. Quality Assurance
Quality checks should be established to review the labeled data. This can involve cross-validation and consensus among multiple annotators to ensure high-quality labels.
6. Iterative Improvement
As models are trained and deployed, feedback loops should be established to continuously improve the labeling process by refining guidelines and methods based on real-world performance analytics.
Choosing the Right Data Annotation Tool
With the growing demand for high-quality labeling training data, selecting an effective data annotation tool is critical. The chosen tool should meet several criteria:
- User-Friendly Interface: A straightforward interface can significantly enhance the productivity of annotators.
- Scalability: The tool should efficiently handle large datasets and support diverse data types.
- Collaboration Features: Facilitate teamwork among data annotators to ensure consistency and quality.
- Integration Capabilities: Ideally, the tool should integrate with other systems used in the AI development pipeline.
- Comprehensive Support: Access to support and resources can help teams address challenges promptly.
The Benefits of Using Keylabs.ai for Labeling Training Data
At Keylabs.ai, we provide a robust data annotation platform designed to cater to the needs of various industries. Our platform offers numerous advantages to help you efficiently label training data:
1. Advanced Technology
Leveraging cutting-edge technologies, our platform enhances the speed and accuracy of data annotation tasks.
2. Expert Annotators
Our team consists of experienced data annotators who understand the nuances of various domains, ensuring high-quality labels tailored to your needs.
3. Customizable Solutions
We provide customizable annotation solutions designed to handle unique data challenges, whether in natural language processing, computer vision, or other AI fields.
4. Continuous Improvement and Feedback
We value feedback and continually strive to improve our processes, ensuring the highest standards of data annotation are maintained.
5. Data Security
At Keylabs.ai, we prioritize your data's privacy and security, employing best practices to safeguard your information throughout the labeling process.
Conclusion: The Future of Labeling Training Data
As AI continues to advance and reshape industries, the importance of effective labeling training data cannot be overstated. It is an essential element that determines the success of various machine learning applications. By employing robust data annotation tools such as those provided by Keylabs.ai, businesses can enhance their AI capabilities and ensure that their models produce accurate, unbiased predictions.
Investing in quality labeling training data is investing in the future of technology. As you navigate your AI journey, remember: the quality of your data will ultimately define the trajectory of your machine learning success.