Unlocking Potential: The Role of Healthcare Datasets for Machine Learning in Business Growth
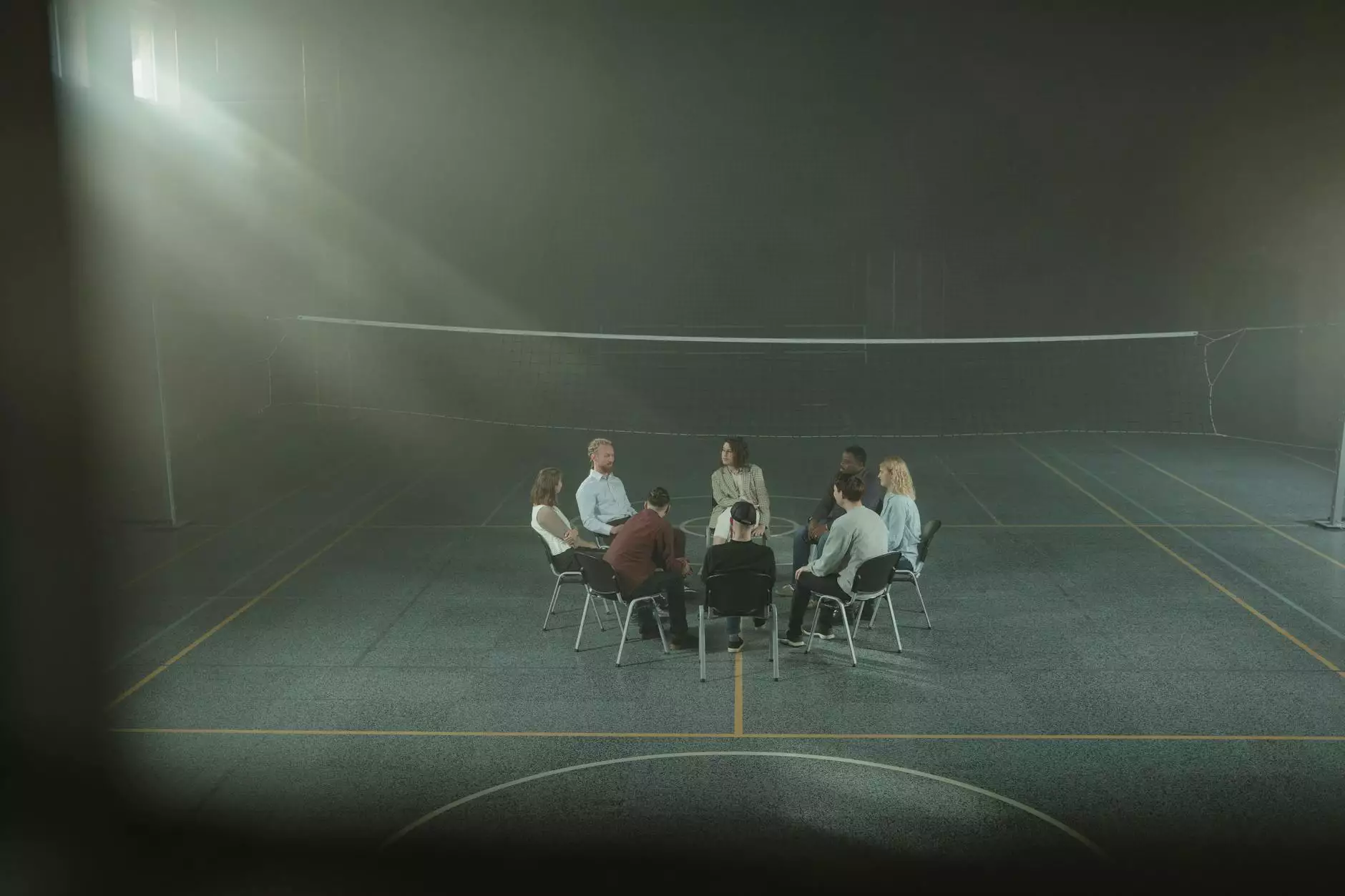
Data is the new oil, especially in the era of digital transformation. Organizations across various industries, particularly in healthcare, are harnessing the power of healthcare datasets for machine learning to drive productivity, enhance service delivery, and generate valuable insights that spur growth. This article explores the profound impact of these datasets on the business landscape, revealing how they can unlock unparalleled opportunities for innovation.
The Power of Data in Healthcare
In the modern business environment, data serves as a cornerstone for decision-making. In healthcare, the proliferation of digital records—ranging from electronic health records (EHRs) to medical imaging—has resulted in a vast reservoir of information. This treasure trove of healthcare datasets for machine learning provides businesses with the tools needed to make informed decisions and adopt data-driven strategies.
Key Components of Healthcare Datasets
- Electronic Health Records (EHRs): Comprehensive patient records that include medical history, medications, allergies, lab results, and more.
- Medical Imaging Data: Data from X-rays, MRIs, and CT scans that aid in diagnostics and predictive modeling.
- Wearable Device Data: Real-time health metrics from devices like fitness trackers and smartwatches.
- Claim and Billing Records: Financial data that reveals patient demographics, service utilization, and more.
- Genomic Data: Information from DNA sequencing that can aid in personalized medicine and targeted treatments.
Machine Learning in Healthcare: An Overview
Machine learning (ML), a subset of artificial intelligence (AI), uses statistical techniques to enable computers to improve their performance on a task through experience. In healthcare, ML algorithms learn from historical data to identify patterns, make predictions, and assist in decision-making processes. By leveraging healthcare datasets for machine learning, businesses can enhance outcomes in various domains, including diagnostics, treatment recommendations, patient management, and operational efficiency.
Benefits of Utilizing Healthcare Datasets
1. Improved Diagnostic Accuracy
Machine learning models trained on extensive healthcare datasets can analyze complex data patterns to assist healthcare professionals in diagnosing conditions with a higher degree of accuracy than traditional methods. This not only aids in early detection of diseases but also reduces the likelihood of misdiagnosis and subsequent costly treatments.
2. Personalized Medicine
The integration of AI and ML into healthcare allows for the development of personalized treatment plans tailored to individual patient characteristics. Using healthcare datasets for machine learning, healthcare providers can analyze genomic data along with lifestyle factors, leading to more effective and targeted therapies.
3. Operational Efficiency
Healthcare organizations can optimize operations and reduce costs by analyzing workflow datasets. By applying machine learning techniques, businesses can forecast patient admissions, manage staffing levels, and streamline resource allocation. Enhanced operational efficiency not only fulfills business objectives but also leads to improved patient experiences.
4. Predictive Analytics for Better Outcomes
Predictive analytics derived from machine learning models can foresee patient outcomes, enabling proactive interventions that enhance care quality. For example, predicting potential readmissions or adverse events allows healthcare teams to take precautionary measures, ultimately improving patient safety and satisfaction.
5. Enhanced Research and Development
Pharmaceutical companies and researchers can leverage healthcare datasets to uncover insights that speed up drug discovery and clinical trials. By analyzing existing patient data, including demographics, genetics, and treatment responses, businesses can identify new drug candidates and optimize trial designs, thus accelerating the pathway to market.
Implementing Machine Learning in Healthcare Businesses
For businesses aiming to implement machine learning solutions using healthcare datasets for machine learning, several steps are crucial:
1. Identify Objectives
Clearly define the business problem you aim to solve. Are you looking to enhance patient diagnostics, optimize resources, or improve treatment outcomes? A well-defined objective guides the selection and application of appropriate ML models.
2. Data Acquisition
Gather relevant datasets that align with your objectives. Ensure that the data is accurate, comprehensive, and ethically sourced, considering patient privacy and data security regulations.
3. Data Cleaning and Preparation
Raw data often contains inconsistencies and missing values. Data preprocessing—cleaning, normalizing, and transforming—is essential to prepare the dataset for machine learning modeling.
4. Select the Right Algorithms
Choose the appropriate machine learning algorithms based on the problem type (regression, classification, clustering) and data characteristics. Popular algorithms in healthcare include decision trees, neural networks, and support vector machines.
5. Model Training and Validation
Train your selected machine learning model using a portion of the dataset while validating it with another subset. This process ensures that your model is not overfitting and can generalize to unseen data.
6. Deployment and Monitoring
After successful validation, deploy the model into operational environments. Continuous monitoring is vital to assess the model’s performance and make adjustments as needed.
Challenges in Utilizing Healthcare Datasets
While the potential benefits of healthcare datasets for machine learning are immense, several challenges must be navigated:
1. Data Privacy and Compliance
Healthcare data is often sensitive, requiring strict adherence to regulations such as HIPAA in the United States. Organizations must implement robust data governance strategies to ensure compliance with legal frameworks.
2. Data Quality Issues
Data inconsistency, incompleteness, and inaccuracy can significantly impede the performance of machine learning models. Continuous efforts toward data quality improvement are essential for reliable outcomes.
3. Integration of Diverse Data Sources
Healthcare data comes from multiple sources. Integrating these varied datasets poses technical and logistical challenges, necessitating sophisticated data engineering strategies.
4. Skill Gaps
The successful implementation of machine learning requires expertise in both healthcare and data science. Companies may face difficulties in attracting and retaining talent skilled in these areas.
Future Trends in Healthcare Machine Learning
The landscape of healthcare and machine learning continues to evolve rapidly. Here are some future trends worth noting:
1. Increased Use of Real-World Evidence
Healthcare datasets are moving beyond clinical trial data to include real-world evidence. The integration of this data will provide a more comprehensive understanding of treatment effects on diverse populations.
2. Enhanced Interoperability
The push for interoperability among healthcare systems will enable seamless data sharing. This will support machine learning initiatives by allowing access to a wider range of datasets for analysis.
3. Advancements in Natural Language Processing (NLP)
As NLP technology improves, the ability to analyze unstructured data from clinical notes, research articles, and patient interactions will expand. This will further enhance the utility of machine learning in healthcare settings.
4. AI-Powered Decision Support Systems
The development of AI-powered clinical decision support systems will provide healthcare professionals with personalized insights at the point of care, augmenting their expertise with machine learning insights.
Conclusion
The potential of healthcare datasets for machine learning to transform business practices in healthcare is profound and expansive. As organizations harness these datasets, they unlock innovative solutions that not only enhance operational efficiency but also significantly improve patient care and outcomes. Embracing machine learning is no longer an option but a necessity for healthcare businesses aiming to thrive in a competitive landscape. The future holds promise for those willing to invest in this transformative journey.
By understanding the importance and applications of healthcare datasets, businesses can position themselves at the forefront of technological advancements, unlocking new avenues for growth, efficiency, and success.